Comparing Machine Learning Models for Predictions in Cloud Dataflow
Pipelines
Integrating for calling TRAINED ML models into the Dataflow
pipeline ( not building a new ml models ) SHOULD CONSIDER Following : Throughput, Latency, Cost, Implementation, Maintenance
Dataflow
Pipelines for Batch Prediction
Batch Prediction
- 전처리된 데이터가 Cloud Storage에 이미 저장되어있는 경우 : AI Platform Batch Prediction Job approach
- AI Platform batch prediction job : TAKES LESS TIME TO PRODUCE PREDICTION for input data, given that the data is already in
Cloud Storage
in the format used for prediction.
- 데이터 처리작업(전처리 후 저장)이 필요한 경우 : Direct model approach + micro-batching
- However, when the batch prediction job is combined with a preprocessing step (extracting and preparing the data from
BigQuery
to Cloud Storage
for prediction) and with a post-processing step (storing the data back to BigQuery
), the direct-model approach produces better end-to-end execution time.
- the performance of the
direct-model
prediction approach can be further optimized using micro-batching.
Summary of Guidelines withExperiments Results
No |
For Batch processing, Guidelines |
Result |
Processing time by 3 approaches depending on 4 different dataset size |
1 |
To Build your batch data processing pipeline, and prediction as part of the pipeline ⇒ USE THE DIRECT-MODEL APPROACH for the BEST PERFORMANCE. |
2 |
To improve the performance of the direct-model approach by creating micro-batches of the data points (MICRO-BATCHING) before calling the local model for prediction to make use of the parallelization of the vectorized operations. |
3 |
Data is populated to Cloud Storage in the format expected for prediction ⇒ USE AI PLATFORM BATCH PREDICTION for the best performance. |
4 |
To use the power of GPUs : Use AI Platform batch prediction |
5 |
Do not use AI Platform online prediction for batch prediction. |
Dataflow
Pipelines for Streaming Prediction
Streaming Prediction
Batch Pipeline - detail
Batch experiments
- Goal : to estimate baby weights
- Data Source : in the Natality dataset in
BigQuery
- ML model :
TensorFlow regression model
.
- For prediction Results :
Cloud Storage
as CSV files
- Data Pipeline :
Dataflow batch pipeline
.
2 approches
Dataflow
with direct-model prediction
Dataflow
with AI Platform batch prediction
Approach 1 - DIRECT-MODEL
Batch Approach 1: Dataflow with direct model prediction |
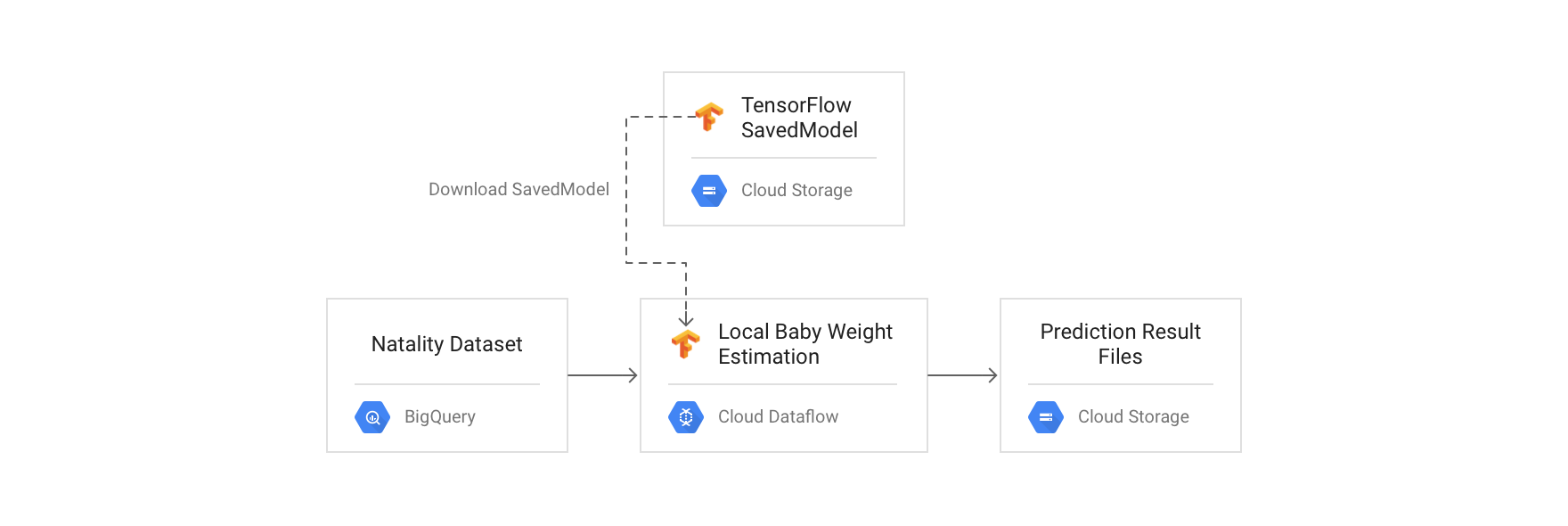 |
Dataflow workers host the TensorFlow SavedModel , called directly for prediction during the batch processing pipeline for each record. |
No calls to remote services (e.g, a deployed model on AI Platform as an HTTP endpoint) : The prediction done locally within each Dataflow worker by using the TensorFlow SavedModel . |
- Read data from
BigQuery
.
- Prepare
BigQuery
record for prediction.
- Call the local TensorFlow
SavedModel
to get a prediction for each record : API call to the deployed model for each record.
- Convert the result (input record and estimated baby weight) to a CSV file.
- Write the CSV file to
Cloud Storage
.
Approach 2 - AI PLATFORM
Approach 2: Dataflow with AI Platform batch prediction |
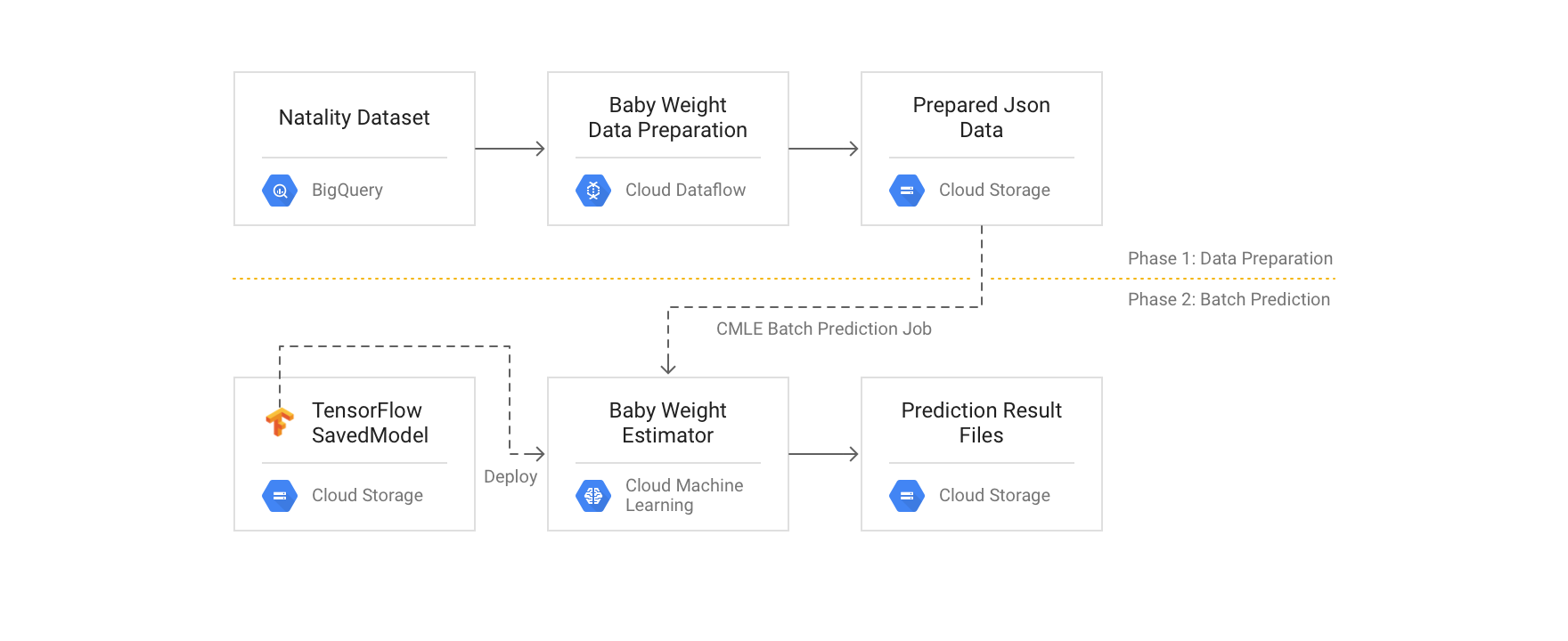 |
TensorFlow SavedModel stored in Cloud Storage → used by AI Platform : Data prepared for prediction and submitted as a batch. |
(1) Data Preparation BigQuery → Dataflow → Cloud Storage |
(2) Batch PredictionAI Platform → Cloud Storage |
(1) BigQuery → Dataflow prepares the data from BigQuery for prediction ⇒ then stores the data in Cloud Storage. ⇒ (2) The AI Platform batch prediction job is submitted with the prepared data, and the prediction results are stored in Cloud Storage. |
- Read data from BigQuery.
- Prepare
BigQuery
record for prediction.
- Write JSON data to
Cloud Storage.
- The
serving_fn
function in the model expects JSON instances as input.
- Submit an
AI Platform batch prediction job
with the prepared data in Cloud Storage
. This job writes the prediction results to Cloud Storage
as well.
DATFLOW - AI PLATFORM PREDICTION JOB
Dataflow
job prepares the data for prediction rather than submitting the AI Platform
prediction job : the data preparation task and the batch prediction task are not tightly coupled.
Cloud Functions
, Airflow
, or any scheduler : orchestrate the workflow by executing the Dataflow
job and then submitting the AI Platform
job for batch prediction.
Streaming Pipeline - detail